Marginal Space Learning for Medical Image Analysis

Medical image analysis is a rapidly growing field that uses computer algorithms to analyze medical images and extract useful information. This information can be used for a variety of purposes, such as diagnosing diseases, planning treatments, and monitoring patient outcomes.
One of the most challenging aspects of medical image analysis is the fact that medical images are often very complex and noisy. This can make it difficult to extract useful information from the images, especially for tasks that require high levels of accuracy, such as diagnosing diseases.
5 out of 5
Language | : | English |
File size | : | 9855 KB |
Text-to-Speech | : | Enabled |
Screen Reader | : | Supported |
Enhanced typesetting | : | Enabled |
Print length | : | 420 pages |
Marginal space learning is a powerful technique that can be used to improve the accuracy of medical image analysis models. Marginal space learning is a type of machine learning that uses a combination of supervised and unsupervised learning to learn from data. Supervised learning involves learning from data that has been labeled with the correct answers, while unsupervised learning involves learning from data that has not been labeled.
Marginal space learning can be used to improve the accuracy of medical image analysis models by learning from both labeled and unlabeled data. Labeled data can be used to learn the relationship between the features in the images and the correct answers, while unlabeled data can be used to learn the underlying structure of the images. This combination of supervised and unsupervised learning allows marginal space learning models to learn more accurate and robust relationships between the features in the images and the correct answers.
Advantages of Marginal Space Learning
There are a number of advantages to using marginal space learning for medical image analysis. These advantages include:
* Improved accuracy: Marginal space learning models have been shown to achieve higher accuracy than traditional machine learning models on a variety of medical image analysis tasks. * Robustness: Marginal space learning models are more robust to noise and other artifacts in the images than traditional machine learning models. * Efficiency: Marginal space learning models can be trained more efficiently than traditional machine learning models.
Disadvantages of Marginal Space Learning
There are also some disadvantages to using marginal space learning for medical image analysis. These disadvantages include:
* Complexity: Marginal space learning models are more complex than traditional machine learning models, which can make them more difficult to implement and interpret. * Computationally expensive: Marginal space learning models can be computationally expensive to train, especially for large datasets.
Applications of Marginal Space Learning in Medical Image Analysis
Marginal space learning has been used in a variety of medical image analysis applications, including:
* Disease diagnosis: Marginal space learning models have been used to diagnose a variety of diseases, including cancer, heart disease, and Alzheimer's disease. * Treatment planning: Marginal space learning models have been used to plan treatments for a variety of diseases, including cancer and heart disease. * Patient monitoring: Marginal space learning models have been used to monitor patient outcomes for a variety of diseases, including cancer and heart disease.
Marginal space learning is a powerful technique that can be used to improve the accuracy of medical image analysis models. Marginal space learning models have been shown to achieve higher accuracy than traditional machine learning models on a variety of medical image analysis tasks. Additionally, marginal space learning models are more robust to noise and other artifacts in the images than traditional machine learning models. However, marginal space learning models are more complex and computationally expensive to train than traditional machine learning models.
5 out of 5
Language | : | English |
File size | : | 9855 KB |
Text-to-Speech | : | Enabled |
Screen Reader | : | Supported |
Enhanced typesetting | : | Enabled |
Print length | : | 420 pages |
Do you want to contribute by writing guest posts on this blog?
Please contact us and send us a resume of previous articles that you have written.
Book
Chapter
Text
Genre
Library
E-book
Magazine
Paragraph
Sentence
Bookmark
Bibliography
Preface
Synopsis
Annotation
Footnote
Manuscript
Codex
Classics
Narrative
Autobiography
Memoir
Reference
Encyclopedia
Thesaurus
Character
Resolution
Archives
Periodicals
Research
Scholarly
Lending
Reserve
Academic
Study Group
Thesis
Awards
Reading List
Book Club
Theory
Textbooks
Paul Kenyon
Marina Budhos
Stephen O Donnell
Salvatore Schiavo Campo
Ronald A Rufo
Cyril Lepeigneux
Alicja Urbanowicz
David Bolton
Dennis Paul Smith
Mark Bowser
Basma Abdel Aziz
Ann E Burg
Carol M Roye
Allan Barry Laboucan
Sincere Jones
Sandra H Scott
Walter Were
Kirkpatrick Hill
Jan Berenstain
Alice Mead
Light bulbAdvertise smarter! Our strategic ad space ensures maximum exposure. Reserve your spot today!
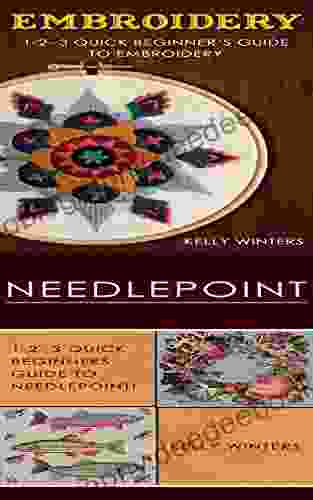

- Roger TurnerFollow ·19.8k
- David PetersonFollow ·6k
- Rex HayesFollow ·14.3k
- Yukio MishimaFollow ·19.6k
- Griffin MitchellFollow ·4k
- Thomas HardyFollow ·12.5k
- Kurt VonnegutFollow ·7.1k
- Graham BlairFollow ·8.2k
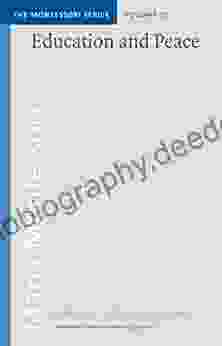

Education And Peace Montessori 10: Where Learning...
A Symphony of Learning and Well-being Amidst...
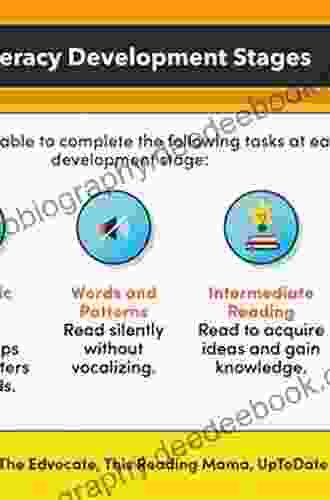

Unveiling the Wonders of Language and Literacy...
Language and literacy...
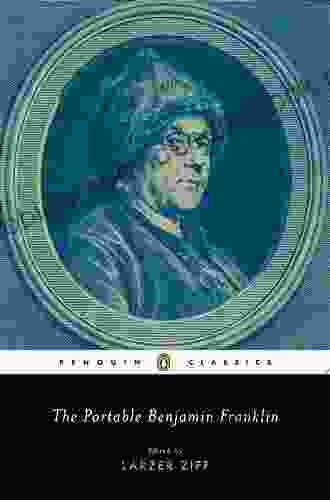

The Portable Benjamin Franklin: A Timeless Collection of...
In the vast tapestry of American history,...
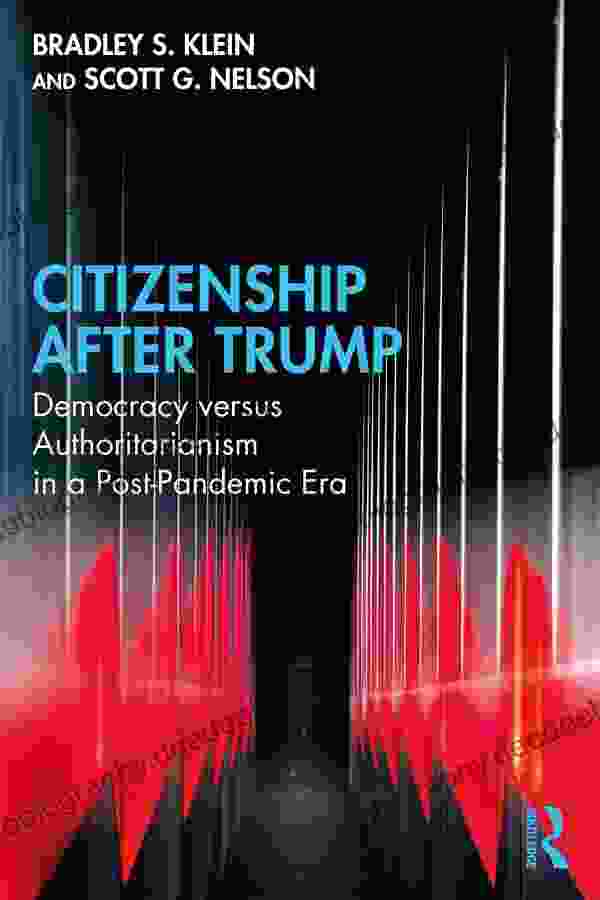

Democracy Versus Authoritarianism in the Post-Pandemic...
The COVID-19...


Get Inspired To Shoot Over 130 Poses
Are you looking for...
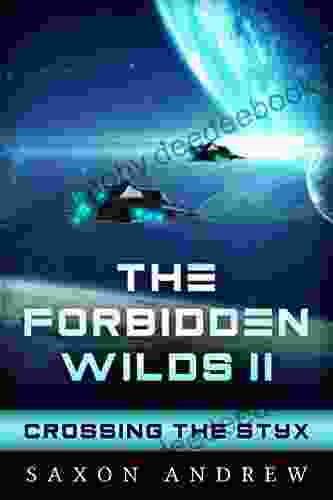

Embark on a Shadowy Journey: The Forbidden Wilds and...
Prologue: A Realm Enshrouded in Darkness As...
5 out of 5
Language | : | English |
File size | : | 9855 KB |
Text-to-Speech | : | Enabled |
Screen Reader | : | Supported |
Enhanced typesetting | : | Enabled |
Print length | : | 420 pages |